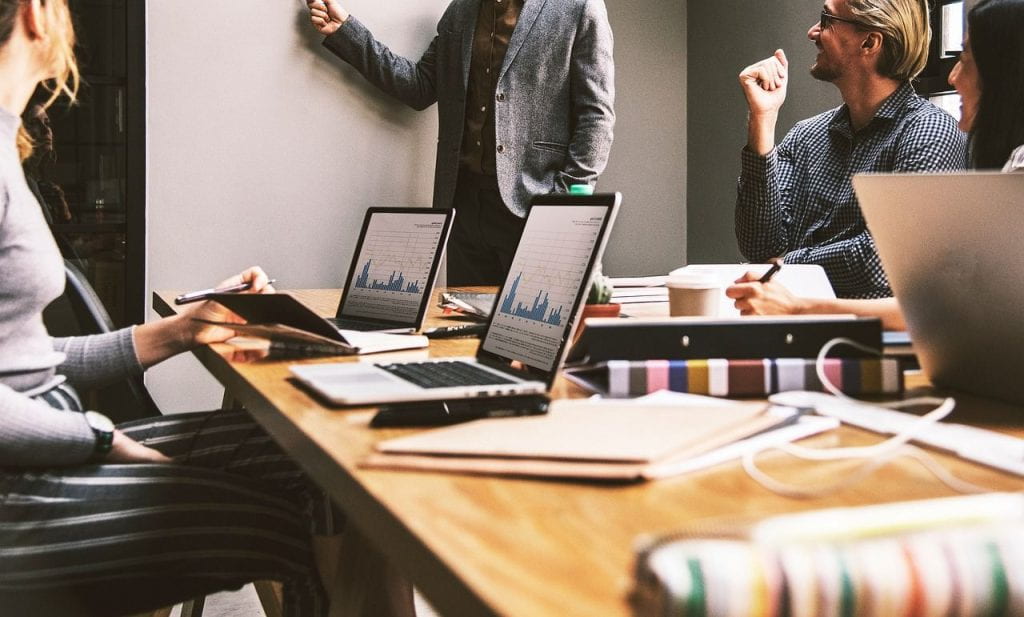
In today’s competitive marketplace, customer retention is a critical focus for businesses. Companies increasingly leverage machine learning (ML) to analyze customer behavior, predict churn, and implement strategies to keep customers engaged. In this blog, we’ll explore a detailed machine learning case study on how AI has been used to improve customer retention, highlighting the role of machine learning projects in shaping a better customer experience.
The Challenge: Customer Churn
Customer churn, or the rate at which customers stop doing business with a company, poses a significant challenge for businesses across industries. Traditional methods of tracking churn often rely on analyzing past data, but they fall short in predicting when or why a customer might leave. This is where machine learning projects become powerful tools. By leveraging vast amounts of data, machine learning algorithms can identify patterns and predict churn more accurately, allowing companies to take proactive measures to retain their customers.
The Solution: Using AI to Predict Churn
In this case study, a subscription-based service provider implemented a machine learning project to tackle the issue of customer retention. The goal was to develop a predictive model that could forecast which customers were most likely to cancel their subscriptions. The company collected historical data on customer behavior, including their purchase history, customer service interactions, engagement levels, and more.
The machine learning project used classification algorithms, such as decision trees and logistic regression, to analyze the data and categorize customers based on their likelihood of churning. This model could predict potential churn weeks in advance, giving the company enough time to take targeted actions, such as offering personalized incentives or addressing service issues.
Good to Read:- Real-World Machine Learning Case Study: How AI is Revolutionizing Healthcare
The Impact: Boosting Retention Rates
After implementing the machine learning-driven predictive model, the company saw a remarkable improvement in its customer retention rates. The AI-powered insights allowed the business to take proactive steps to keep high-risk customers engaged. For example, customers who showed signs of potential churn were offered tailored discounts, loyalty programs, or specialized customer support. These timely interventions made a significant difference, reducing churn rates by 25%.
The machine learning project also helped the company identify patterns that led to churn, such as reduced customer interactions over time or dissatisfaction with specific services. With this knowledge, the company made broader improvements in their offerings, leading to higher overall customer satisfaction.
Key Takeaways from the Case Study
This machine learning case study demonstrates the incredible potential AI holds in improving customer retention. The ability to predict churn with accuracy empowers businesses to make data-driven decisions and maintain stronger customer relationships. By investing in ML projects, companies can better understand their customers, take proactive actions, and ultimately reduce churn rates.
Good to Read:- Machine Learning Case Study: Predictive Analytics in E-Commerce
Conclusion
As demonstrated in this case study, machine learning projects focused on customer retention can deliver significant business benefits. The predictive power of machine learning allows companies to anticipate customer needs, prevent churn, and ensure a higher level of engagement. In an increasingly data-driven world, leveraging AI for customer retention is not only a competitive advantage but also a necessity for long-term success.